Part 2: Physical AI in Manufacturing: What It Takes to Make It Work
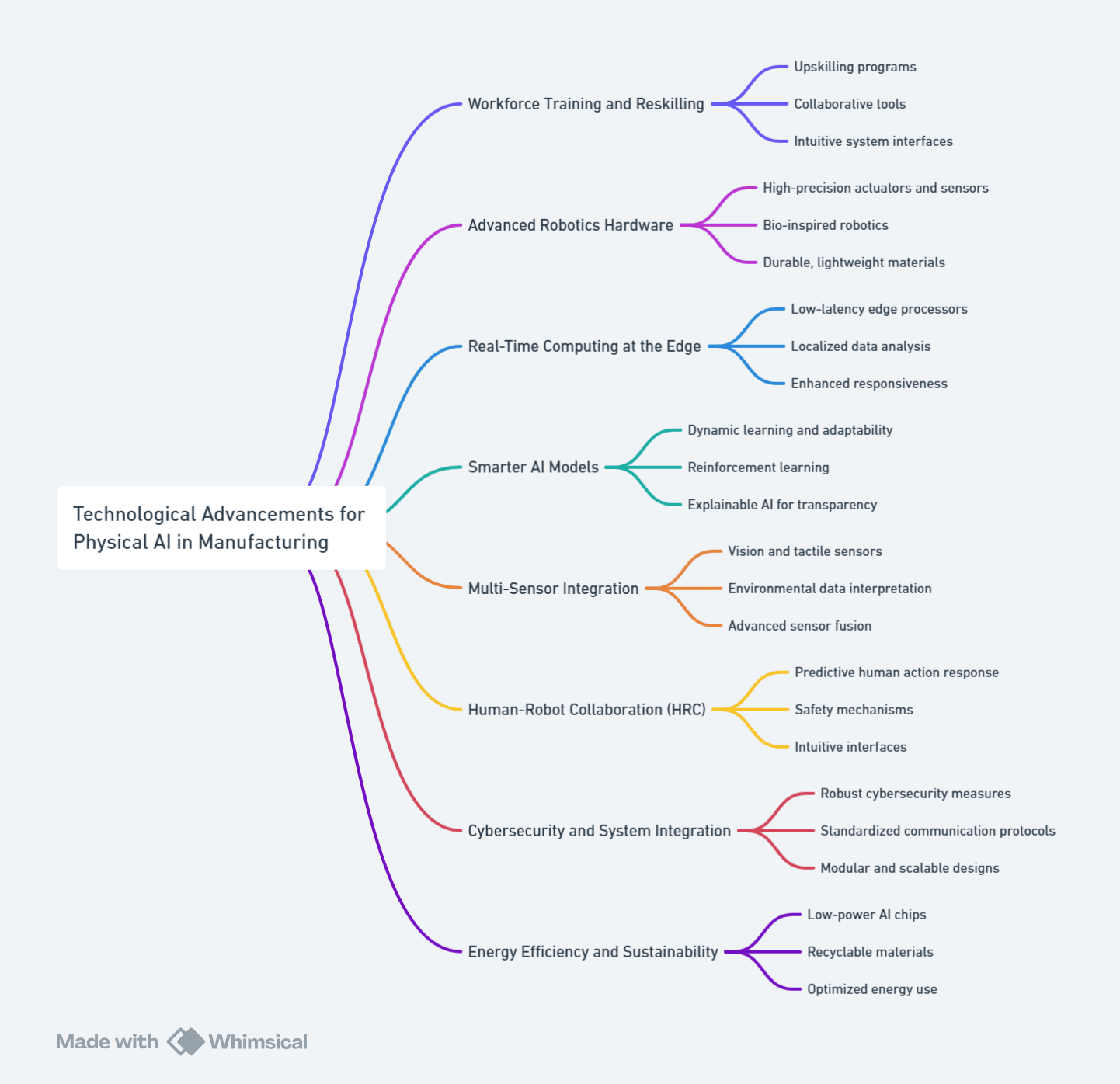
Physical AI is on the rise, promising safer, more adaptive, and more efficient manufacturing. But let’s be real—adopting it isn’t just about installing a few smart robots and calling it a day. To make Physical AI work at scale, businesses need the right mix of technology, workforce readiness, and support structures. It’s not a one-size-fits-all solution, either. Adoption will vary based on factors like process complexity, compliance requirements, safety considerations, and overall necessity.
Key Takeaways:
✅ Empowering the Workforce – It’s not just about machines; it’s about people. Training, intuitive interfaces, and seamless human-robot collaboration will be key to ensuring technology enhances human talent.
✅ Prioritizing Sustainability and Security – AI-driven manufacturing must be energy-efficient, environmentally conscious, and cyber-secure. Without these priorities, widespread adoption will stall, not creating the ecosystem needed for further development.
Here’s what’s needed to make Physical AI a practical, sustainable, and scalable reality in modern manufacturing.
1. Workforce Training and Reskilling: The Human Element Matters
Talent comes first. If people don’t know how to work alongside AI-driven systems, adoption won’t stick. Physical AI is about augmenting our skills. Workers need training to operate, monitor, and maintain AI-driven systems, and AI needs to understand human workflows to be useful. Intuitive tools, collaborative platforms, and structured upskilling programs will be critical.
2. Smarter, More Capable Robotics
Physical AI needs hardware that can actually handle real-world manufacturing environments. This means:
High-precision actuators and sensors – Robots that can make fine adjustments on the fly.
Bio-inspired robotics – Think soft grippers and flexible automation that can handle delicate or irregular objects.
Lightweight, durable materials – To improve efficiency and longevity while reducing energy consumption.
3. Real-Time Edge Computing: AI Where It’s Needed
AI-driven machines need to process vast amounts of data—fast. Relying on cloud computing alone introduces latency, which can be a deal breaker on the factory floor. Edge computing, with ultra-low-latency AI processors, will allow AI to analyze and act on data locally, making systems more responsive and reliable.
4. Smarter AI Models: Adaptability Is Key
For Physical AI to be truly effective, its machine learning models must be able to adapt to new tasks and environments.
Reinforcement learning will help machines optimize performance over time.
Explainable AI will be necessary for compliance, safety, and trust in AI-driven decision-making.
5. Multi-Sensor Integration: Seeing, Feeling, Understanding
AI systems need multiple data sources to make informed decisions. This means:
Vision and tactile sensors – AI needs to see and “feel” its environment.
Environmental sensors – Detecting heat, humidity, and pressure for better process control.
Sensor fusion technology – Integrating all these inputs so machines can operate with real-world awareness.
These advancements will allow AI-driven systems to react intelligently to their surroundings rather than simply following pre-programmed routines.
6. Human-Robot Collaboration: A Safer, Smarter Partnership
Physical AI should make it more intuitive for workers and robots to operate in the same space. That means robots need to:
Predict human actions to avoid collisions and improve workflow.
Have robust safety mechanisms to prevent workplace accidents.
Feature intuitive interfaces like voice commands and gesture recognition.
7. Cybersecurity & System Integration: Protecting AI-Driven Factories
More AI means more connectivity, and that means greater cybersecurity risks. To protect sensitive data, systems, assets and workers, manufacturers need:
Robust cybersecurity protocols that prevent breaches and attacks.
Standardized communication frameworks that allow seamless system integration.
Modular designs so AI-driven systems can be easily updated without overhauling entire factories.
Without strong security, AI in manufacturing becomes a liability rather than an asset.
8. Energy Efficiency & Sustainability:
AI-driven manufacturing must be energy-efficient and environmentally responsible. This means:
Low-power AI chips that minimize energy consumption.
Recyclable materials in robotic components to reduce waste.
Optimization algorithms that ensure operations are running at peak efficiency.
Physical AI must factor in more ways to contribute to the circular economy, beyond the standards of energy efficiency, recyle, reuse and renewable resources
The Bottom Line: Physical AI Needs More Than Just Hype
AI-driven manufacturing isn’t a sci-fi fantasy—it’s happening now. But to fully unlock its potential, businesses need to prioritize workforce readiness, smarter hardware, real-time AI, better security, and sustainable practices. It is critical that these tools, solutions and practices are accessible and available to all sizes of manufacturers. That involves business models, modular solutions and support structure. We will explore this in the next blog.