How Digital Twins Are Transforming Cost Estimation in Manufacturing
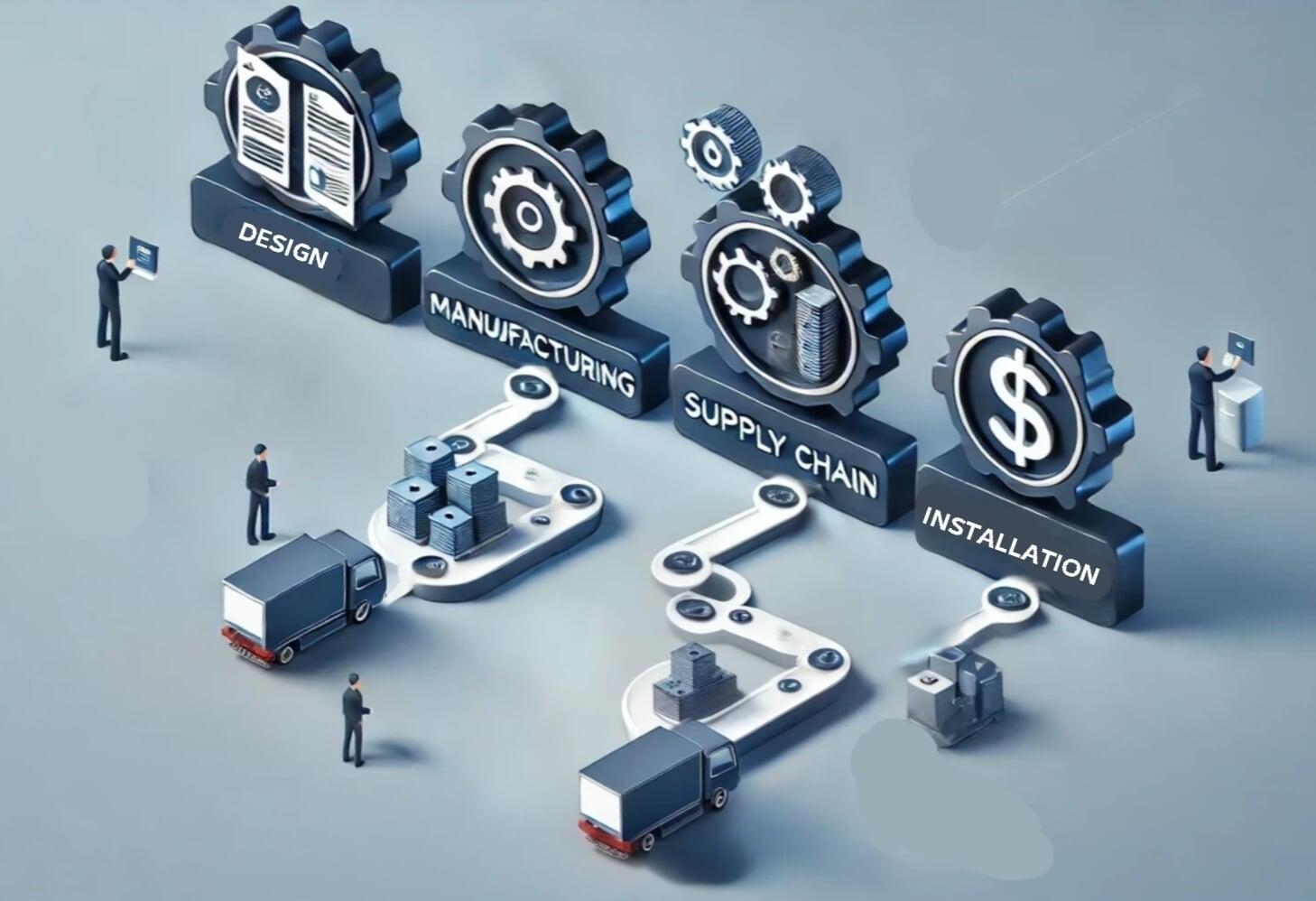
Industry: Automotive; General Engineering
Areas Addressed: Digital Twins
Capabilities: Digital Twin in cost estimation and cost reductionSummary
This blog explores how digital twins enhance cost estimation in automation projects, using the SCARA robotic automation case study. By integrating digital twins, the company was able to simulate, validate, and optimize cost factors before physical deployment, leading to reduced financial risks, increased efficiency, and better decision-making.
The blog details how digital twins were leveraged in various cost categories, including material and equipment costs, workforce planning, operational costs, compliance, and lifecycle maintenance. While some aspects were fully utilized, others were partially explored, offering insights into future opportunities.
Key Takeaways
✅ More Accurate Cost Estimation: Digital twins helped refine material costs, labor allocation, and energy use, reducing errors and waste.✅ Improved Workforce Planning: Simulated labor shifts and training needs to ensure better allocation and workforce upskilling.
✅ Optimized Production & Operations: Reduced cycle time, improved machine efficiency, and cut unnecessary movements.
✅ Better Supply Chain Visibility: Provided procurement and lead time insights, improving cash flow management.
✅ Compliance & Safety Cost Reductions: Identified safety risks early, reducing factory acceptance test (FAT) failures and deployment delays.
✅ Lifecycle & Maintenance Cost Planning: Enhanced maintenance scheduling, preventing unexpected downtime and improving long-term scalability.
Using Digital Twins in Cost Estimation
Digital twins have emerged as a powerful tool in manufacturing, enabling companies to simulate, validate, and optimize processes before physical implementation. On Feb 17, I shared a case study illustrating how digital twins were used to define solutions, communicate requirements, and create essential assets for both customers and solution builders. This discussion led to further exploration of their role in cost estimation. While we leveraged digital twins in some aspects of cost estimation, there are additional areas to explore.
The case study focused on implementing a SCARA robotic automation system to address bottlenecks in an induction hardening process. By integrating a digital twin, the company effectively reduced design iterations, improved efficiency, and mitigated deployment risks.
In this blog, we will break down how digital twins contribute to a structured cost estimation framework in automation projects, some of which we used partially:
1. Material & Equipment Cost Estimation
The digital twin modeled the SCARA robot, grippers, and custom pallet system to determine the optimal design for handling parts efficiently.
It simulated the frame and safety enclosures, ensuring materials, layout, access were optimized.
It provided a design bill of material, which was used to estimate material costs internally and with suppliers.
✅ Cost Insight:
Cost estimation was more accurate.
Interference errors, typically tested in physical prototypes, were addressed digitally, reducing waste and time to market.
2. Manufacturing Cost Estimation at Our Factory (Partially Used)
Digital Twin models were based on CAD drawings, which were used for CAM machining estimates.
CAD drawings and CAM software were used to estimate manufacturing costs for internally machined parts, covering machine operations, tooling, changeover, process time, and material cost.
The assembly team used the digital twin simulation and CAD to estimate assembly skills needed, time assembly time, quality parameters, and testing protocols.
✅ Cost Insight:
Greater accuracy in machining estimates and scheduling of parts in the machine shop.
The factory team gained data-driven visibility into scheduling, resource allocation, and coordination with planning and procurement.
3. Operational Costs at Client Site
The digital twin optimized the SCARA robot’s motion path, reducing cycle time.
While power consumption was not modeled, it can be incorporated where energy is a significant input in material conversion and part of the company's sustainability goals.
✅ Cost Insight:
Improved throughput by 20%.
4. Workforce Planning & Labor Cost Estimation
The digital twin simulated robot cycle time and manual operator workload to quantify labor savings.
It predicted how job roles would shift, determining training costs for upskilling operators and maintenance teams.
✅ Cost Insight:
The robotic system reduced manual labor by 1.5 operators per shift, allowing skilled operators to be deployed in other areas.
Trained and upskilled one maintenance technician for robot operations and preventive maintenance.
5. Supply Chain & Logistics Cost Estimation (Partially Used)
A full supply chain digital twin was not available; instead, inventory, planning and purchase modules were used.
When manufacturing operations and supply chain are well-integrated, supply chain management tools can be used to simulate planning, procurement, scheduling, execution, and what-if scenarios.
Through MRP, alternative sourcing was evaluated based on lead times.
✅ Cost Insight:
Cost estimates and lead times were fine-tuned, providing the finance team with cash flow visibility.
6. Compliance & Safety Indirect Cost Reduction (Partially Used)
The digital twin modeled safety scenarios, ensuring compliance and adherence to safety standards.
It simulated operator interactions, validating the effectiveness of lockout/tagout (LOTO) procedures.
✅ Cost Insight:
Improved worker safety metrics for the factory team.
Reduced factory acceptance testing (FAT) failures by addressing issues in the simulation.
Rejection at automation FAT typically costs the provider 6-10 weeks of delays and additional material costs, eroding margins.
7. Lifecycle & Maintenance Cost Estimation (Partially Used)
The digital twin predicted robotic maintenance schedules, optimizing spare part inventory.
Simulated how automation impacted the entire production flow, ensuring the system remained scalable.
If and when mission-critical, component **wear rates **can be modeled to plan for future upgrades.
✅ Cost Insight:
Proactively planned for spare part replacements, training, and support assets, reducing unplanned downtime.
Identified a preventive maintenance strategy, increasing productivity and adoption.
Final Cost Optimization Impact
By leveraging the digital twin for cost estimation, the company:
✔ Reduced upfront material costs by 15%.
✔ Optimized labor savings while ensuring workforce adaptability.
✔ Improved compliance and safety metrics for factory stakeholders.
✔ Planned preventive maintenance, reducing unplanned downtime.
✔ Improved adoption and automation experience through digital assets.
💡 Overall, digital twins optimized cost estimation, reducing financial risk and improving ROI before physical deployment.